棒グラフは、種別、地域別などのカテゴリーで分けられるデータの特徴を示すグラフである。Python では を利用して、棒グラフを描くことができる。
基本的な棒グラフの作成方法
棒グラフは matplotlib の bar
メソッドを利用して作成する。x 軸座標(あるいは項目名)と y 軸座標(値)を用意して、bar(x, y)
へ代入するだけで、棒グラフが作成される。x 軸が数字ではなく、項目名となっているとき、bar(x, y)
のままでグラフを描くと、項目名がアルファベット順にソートされる。これを防ぐために、x 軸が項目名のときは、一時的に数値 x_position
に直して棒グラフを描き、bar
の tick_label
オプションで、x 軸座標の数値と項目名に入れ替える。
import matplotlib.pyplot as plt
import seaborn as sns
import numpy as np
sns.set()
sns.set_style('whitegrid')
sns.set_palette('gray')
x = np.array(['ERS1', 'ERS2', 'ETR1', 'ETR2', 'EIN4'])
y = np.array([12.0, 3.1, 11.8, 2.9, 6.2])
x_position = np.arange(len(x))
fig = plt.figure()
ax = fig.add_subplot(1, 1, 1)
ax.bar(x_position, y, tick_label=x)
ax.set_xlabel('gene')
ax.set_ylabel('gene expression [log(TPM)]')
fig.show()
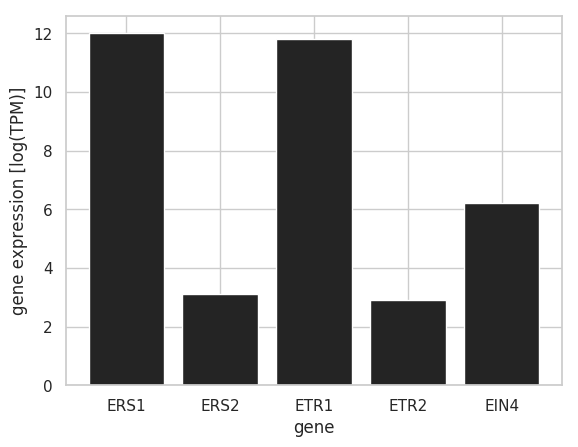
x 軸のラベルが長い場合、重なることがある。このとき、set_xtickslabels
メソッドを使用して、座標軸のラベルを回転させることができる。
fig = plt.figure()
ax = fig.add_subplot(1, 1, 1)
ax.bar(x_position, y)
ax.set_xticks(x_position)
ax.set_xticklabels(x, rotation=15)
plt.show()
横向きの棒グラフは barh
メソッドを利用する。オプションは、bar
と同様に使える。
import matplotlib.pyplot as plt
import seaborn as sns
import numpy as np
sns.set()
sns.set_style('whitegrid')
sns.set_palette('gray')
x = np.array(['ERS1', 'ERS2', 'ETR1', 'ETR2', 'EIN4'])
y = np.array([12.0, 3.1, 11.8, 2.9, 6.2])
x_position = np.arange(len(x))
fig = plt.figure()
ax = fig.add_subplot(1, 1, 1)
ax.barh(x_position, y, tick_label=x)
ax.set_ylabel('gene')
ax.set_xlabel('gene expression [log(TPM)]')
plt.show()
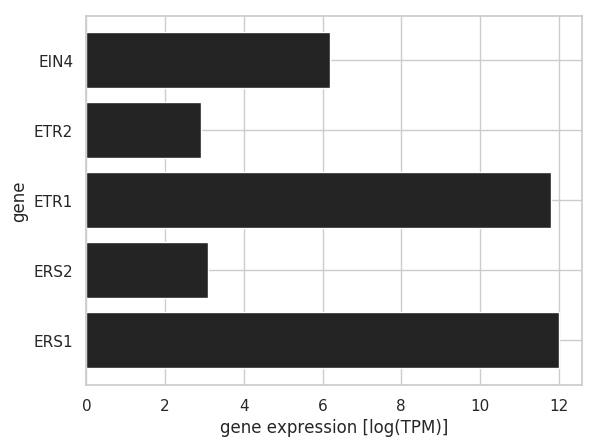
並列表示の棒グラフ
2 本の棒グラフを並べて描くとき、plotly.bar
を 2 回実行すればよい。ただし、2 本の棒グラフの幅をそれぞれ指定あげる必要がある。例えば、棒グラフの幅を 0.4 にすると、2 本の棒グラフの合計幅が 0.8 となる。項目と項目の間に 0.2 の余白が作られる。また、2 本目の棒グラフの x 座標は、1 本目の棒グラフの右に描くために、x 座標を調整する必要がある。例えば、棒グラフの幅が 0.4 のとき、2 本目の棒グラフの x 座標を全体的に +0.4 側へシフトする。
import matplotlib.pyplot as plt
import seaborn as sns
import numpy as np
sns.set()
sns.set_style('whitegrid')
sns.set_palette('Paired')
x = np.array(['ERS1', 'ERS2', 'ETR1', 'ETR2', 'EIN4'])
x_position = np.arange(len(x))
y_control = np.array([12.0, 3.1, 11.8, 2.9, 6.2])
y_stress = np.array([6.2, 3.4, 6.8, 2.0, 6.8])
fig = plt.figure()
ax = fig.add_subplot(1, 1, 1)
ax.bar(x_position, y_control, width=0.4, label='control')
ax.bar(x_position + 0.4, y_stress, width=0.4, label='stress')
ax.legend()
ax.set_xticks(x_position + 0.2)
ax.set_xticklabels(x)
plt.show()
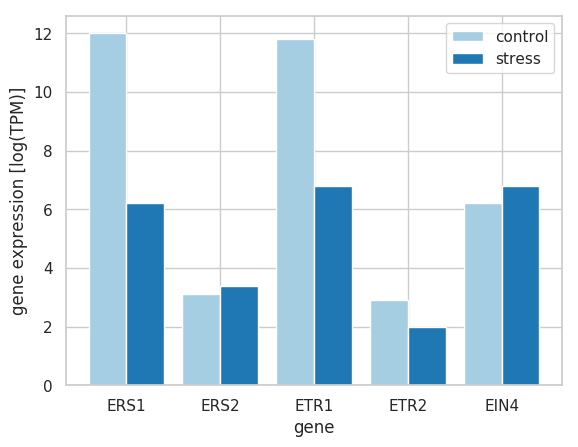
積み上げ棒グラフ
積み上げ棒グラフも bar
を 2 回実行すればよい。ただし、2 本目の棒グラフをどこから積み上げあるのかを bottom
オプションで指定してあげる必要がある。
import matplotlib.pyplot as plt
import seaborn as sns
import numpy as np
sns.set()
sns.set_style('whitegrid')
sns.set_palette('Paired')
x = np.array(['sample 1', 'sample 2', 'sample 3'])
x_position = np.arange(len(x))
y_DEG2 = np.array([220, 230, 260])
y_DEG1 = np.array([810, 940, 870])
fig = plt.figure()
ax = fig.add_subplot(1, 1, 1)
ax.bar(x_position, y_DEG1, label='FDR < 0.01')
ax.bar(x_position, y_DEG2, bottom=y_DEG1, label='0.01 <= FDR = 0.05')
ax.legend()
ax.set_xticks(x_position + 0.2)
ax.set_xticklabels(x)
ax.set_xlabel('sample')
ax.set_ylabel('#DEGs')
plt.show()
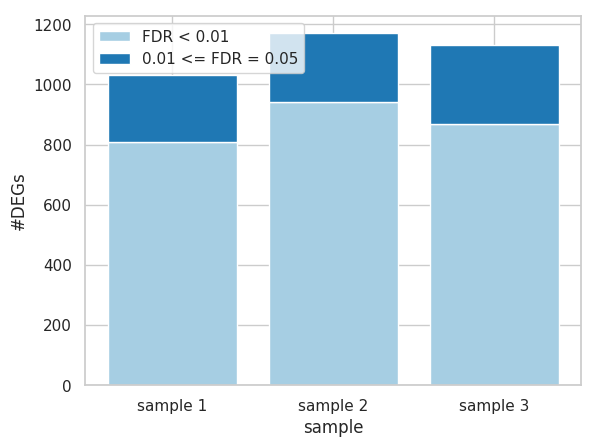
エラーバー付き棒グラフ
棒グラフを描くメソッド bar
に yerr
オプションがある。このオプションにあらかじめ計算した標準偏差あるいは誤差を代入すると、棒グラフとともにエラーバーも表示される。yerr
を指定するだけだと、エラーバーは縦棒として表示される。エラーバーを「工」の形にするためには、capthick
と capsize
を 0 よりも大きい値に指定する必要がある。
import matplotlib.pyplot as plt
import seaborn as sns
import numpy as np
sns.set()
sns.set_style('whitegrid')
sns.set_palette('gist_yarg')
x = np.array(['ERS1', 'ERS2'])
x_position = np.arange(len(x))
ers1 = np.array([12.1, 10.9, 11.1, 12.9])
ers2 = np.array([8.6, 7.9, 10.2, 6.2])
ers1_mu = ers1.mean()
ers2_mu = ers2.mean()
ers1_sd = ers1.std()
ers2_sd = ers2.std()
y = np.array([ers1_mu, ers2_mu])
e = np.array([ers1_sd, ers2_sd])
error_bar_set = dict(lw = 1, capthick = 1, capsize = 20)
fig = plt.figure()
ax = fig.add_subplot(1, 1, 1)
ax.bar(x_position, y, yerr = e,
tick_label=x,
error_kw=error_bar_set)
plt.show()
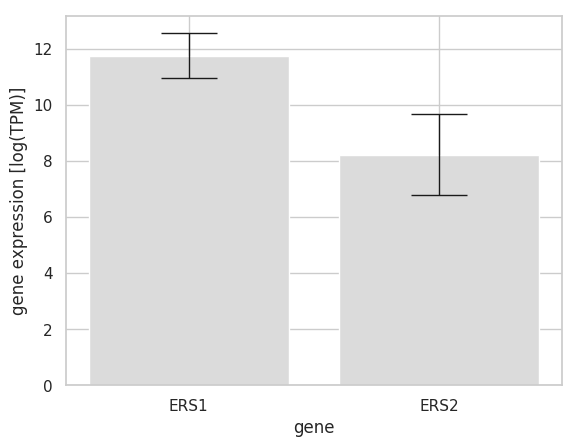
エラー付き棒グラフの上に jitter plot を重ねることもできる。このとき、上述のように棒グラフを描いてから、seaborn ライブラリーの stripplot
メソッドを使用して jitter plot を描く。
import matplotlib.pyplot as plt
import seaborn as sns
import numpy as np
import pandas as pd
plt.style.use('default')
sns.set()
sns.set_style('whitegrid')
sns.set_palette('gist_yarg')
np.random.seed(2018)
df = pd.DataFrame({
'ERF1': np.random.normal( 6, 2.1, 10),
'ERF2': np.random.normal(12, 2.2, 10),
'ERF3': np.random.normal(18, 2.5, 10),
})
fig = plt.figure()
ax = fig.add_subplot(1, 1, 1)
# bar chart & error bar
x_position = np.arange(len(df.columns))
error_bar_set = dict(lw = 1, capthick = 1, capsize = 20)
ax.bar(x_position, df.mean(), yerr=df.std(), tick_label=df.columns, error_kw=error_bar_set)
# jitter plot
df_melt = pd.melt(df)
print(df_melt.head())
## variable value
## 0 ERF1 5.418788
## 1 ERF1 7.221887
## 2 ERF1 10.511638
## 3 ERF1 3.313077
## 4 ERF1 7.054781
sns.stripplot(x='variable', y='value', data=df_melt, jitter=True, color='black', ax = ax)
ax.set_xlabel('gene')
ax.set_ylabel('gene expression [log(TPM)]')
plt.show()
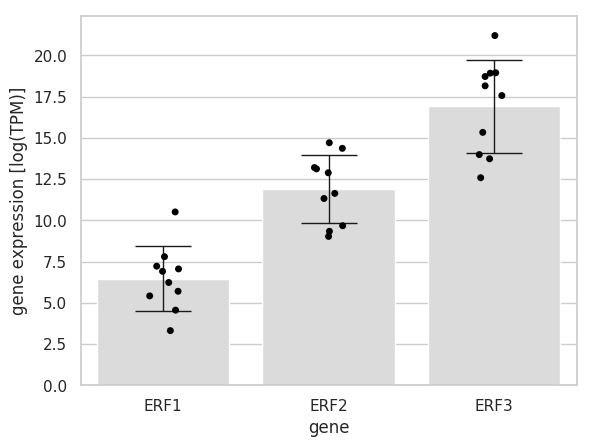